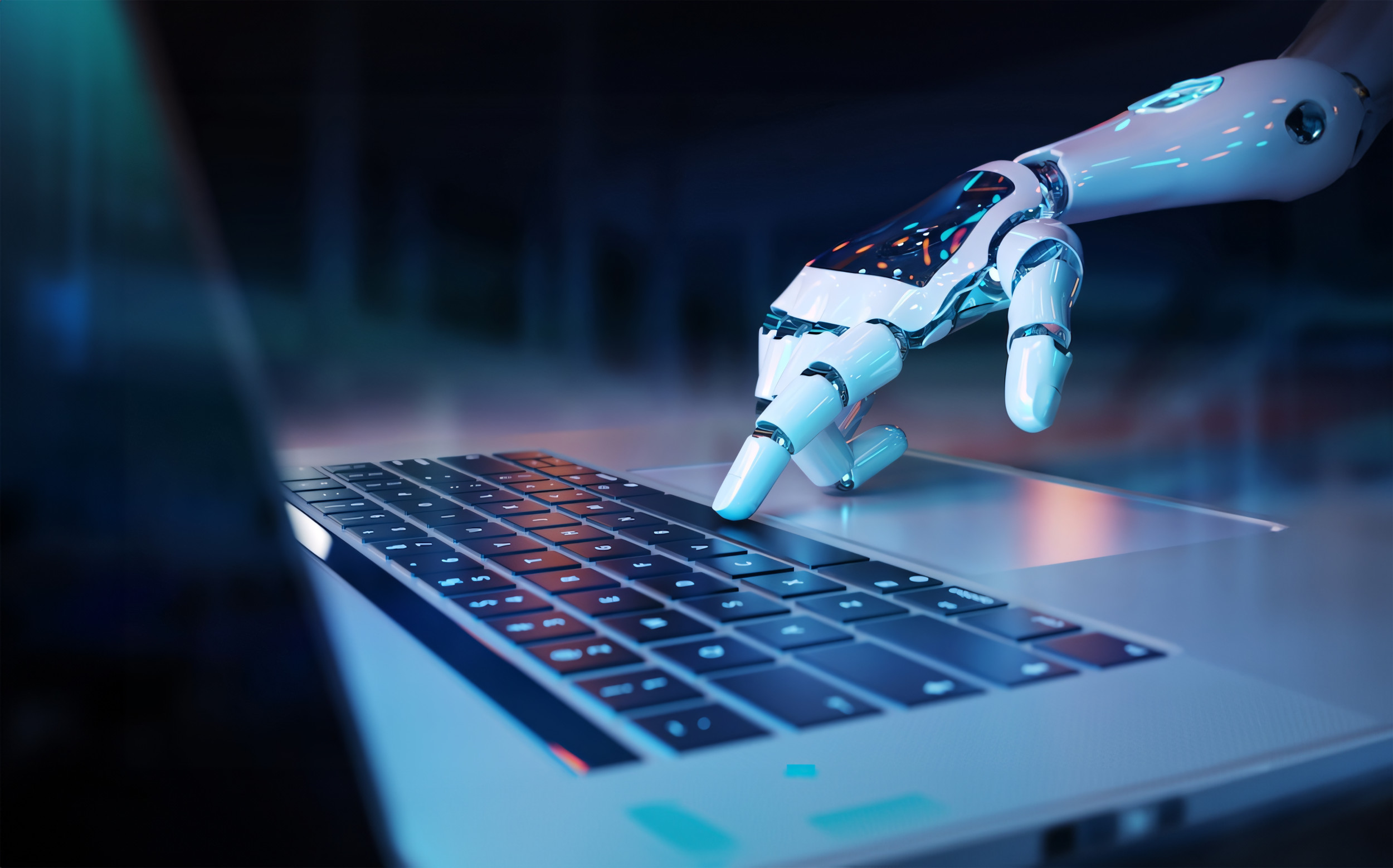
When we speak of 'AI', our minds today gravitate towards the growing list of powerful models that providers like OpenAI and Meta have brought to center stage over the past 12 months. We know AI and LLMs can fundamentally transform modern business as we know it, but many obstacles are still present. At the end of the day, as the infamous AI expert Andrew Ng reminds us, 'AI = Model + Data.'
The past 15 years of AI breakthroughs have been weighted far more heavily towards the 'model' component of this AI equation, and with the recent widespread adoption of large language models (LLMs), companies now find themselves gifted with some of the most powerful algorithms we have ever seen. But what about the data?
A culinary analogy is helpful here: Let's say that 'Meal = Chef + Ingredients.' The current breakthroughs in AI that we are witnessing are akin to some serious upskilling of the chef. But, no matter how high-performing this chef becomes, problems that exist in the raw ingredients themselves will always impair the resulting quality of the meal prepared. The same goes for data.
As enterprises in 2023 race to get ahead of the Generative AI (GenAI) adoption curve, it is becoming clear that most are indeed very far from having the right data foundation in place to support the reliable adoption of LLMs, particularly in a scalable way.
Whilst quality and controls around structured data (e.g., numerical tables of data) have always been a top-of-mind subject for data leaders, the rise of LLMs in 2022 has meant that, for the first time, unstructured data can be leveraged for countless AI applications. Unstructured data refers to anything from text-based documents like contracts, policies or market reports, to files such as videos, images and audio recordings. Such data is the core ingredient for the plethora of chatbots, smart knowledge assistants and content-generation engines that companies are eager to deploy, and yet has rarely received any attention from a data governance perspective.
The familiar scene for unstructured data in most companies looks like thousands upon thousands of documents spread far and wide across every corner of the organization, from file-sharing systems like Dropbox and SharePoint to clusters of data homed in the cloud to myriad documents gathering dust in legacy systems or local folders. A historic lack of systematic management of such data is creating three big challenges that must be addressed to unlock reliable usage of AI: data relevance, data quality and data safety.
Data Relevance
LLMs are intelligent but need a lot of hand-holding in order to sift through thousands of documents and select the most relevant source to infer an answer from. Take an insurance company that has deployed a knowledge assistant trained on the masses of insurance policies they possess. Given that policies vary in subtle ways based on anything from geography to accident type to customer profile, it is very challenging to ensure that an answer returned from the knowledge assistant will have been retrieved from a document that reflects the exact contextual knowledge of a given question.
Data Quality
Data quality in the context of unstructured data is still a highly unexplored subject. Standard approaches for assessing outliers, freshness, completeness, etc., of tabular datasets no longer hold true in this new paradigm, but the same problems exist.
What happens if I ask my chatbot to tell me something about "CompanyX," but the ideal dataset to use actually has the naming convention "CompX?" What happens when I have conflicting information across many documents? What if the sea of data I am feeding into a model contains outdated reports or those will become obsolete over time?
Data Safety
Last but by no means least is the subject of data safety. Companies require measures to ensure that sensitive information, ranging from Personal Identifiable Information (PII) to proprietary data and IP, cannot be exposed to models.
Take GDPR in Europe: a company could spend millions of dollars training its own proprietary customer support model and unknowingly include a document containing a customer's address. Should this customer request for their data to be removed, then the organization would have no choice but to delete the entire model.
Beyond external data sharing, many companies are also struggling to maintain data access controls within the organization itself. This is particularly true for knowledge assistants or chatbots, where enforcing guardrails around which employees can access which pieces of data via the model is a big sticking point.
So What Can Companies Do?
Organizations serious about adopting LLMs require a clear strategy for their unstructured data that addresses the three areas above. They can work on these challenges in-house, which often is a heavy investment both in time and money. However, a new crop of highly skilled companies are coming to market with true industry expertise in these areas.
There are a number of new companies addressing this problem with deep expertise, so let's take a look at a couple that are doing innovative work in the space. Deasie is a data governance startup built by former McKinsey/QuantumBlack employees who focus on intelligently tagging thousands of documents across a company with key themes, including document purpose, version, and the types of PII present. Another example is Kobalt Labs, which built a model-agnostic API that anonymizes and replaces PII and other sensitive data from structured and unstructured input, allowing enterprises to use third-party models like ChatGPT safely and securely.
With the pace of advancement in the world of GenAI, it is tempting to feel that companies are on the brink of a wide-scale AI transformation. But, whilst these models offer a tremendous opportunity, any meaningful progress towards the adoption of this technology must be accompanied by a robust and thoughtful data management strategy. Data governance is coming out of the Trough of Disillusionment of the Gartner hype cycle and the enterprises that get ahead of their data governance now can be winners in the long run.
Uncommon Knowledge
Newsweek is committed to challenging conventional wisdom and finding connections in the search for common ground.
Newsweek is committed to challenging conventional wisdom and finding connections in the search for common ground.
About the writer
To read how Newsweek uses AI as a newsroom tool, Click here.